Modern Graph Theory Algorithms with Python: Harness the Power of graph algorithms ...
Lun Jul 29, 2024 6:25 pm
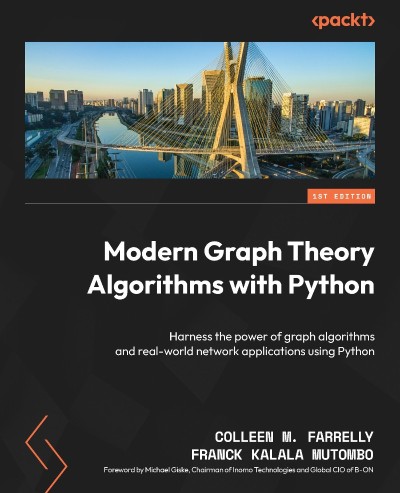
[/center]
pdf | 35.75 MB | English | Isbn:9781805120179 | Author: Colleen M. Farrelly, Franck Kalala Mutombo, Michael Giske (Foreword by) | Year: 2024
[/center]
About ebook: Modern Graph Theory Algorithms with Python: Harness the power of graph algorithms and real-world network applications using Python
Solve challenging and computationally intensive analytics problems by leveraging network science and graph algorithms
• Learn how to wrangle different types of datasets and analytics problems into networks
• Leverage graph theoretic algorithms to analyze data efficiently
• Apply the skills you gain to solve a variety of problems through case studies in Python
• Purchase of the print or Kindle book includes a free PDF eBook
We are living in the age of big data, and scalable solutions are a necessity. Network science leverages the power of graph theory and flexible data structures to analyze big data at scale. This book guides you through the basics of network science, showing you how to wrangle different types of data (such as spatial and time series data) into network structures. You'll be introduced to core tools from network science to analyze real-world case studies in Python. As you progress, you'll find out how to predict fake news spread, track pricing patterns in local markets, forecast stock market crashes, and stop an epidemic spread. Later, you'll learn about advanced techniques in network science, such as creating and querying graph databases, classifying datasets with graph neural networks (GNNs), and mining educational pathways for insights into student success. Case studies in the book will provide you with end-to-end examples of implementing what you learn in each chapter. By the end of this book, you'll be well-equipped to wrangle your own datasets into network science problems and scale solutions with Python.
• Transform different data types, such as spatial data, into network formats
• Explore common network science tools in Python
• Discover how geometry impacts spreading processes on networks
• Implement machine learning algorithms on network data features
• Build and query graph databases
• Explore new frontiers in network science such as quantum algorithms
If you're a researcher or industry professional analyzing data and are curious about network science approaches to data, this book is for you. To get the most out of the book, basic knowledge of Python, including pandas and NumPy, as well as some experience working with datasets is required. This book is also ideal for anyone interested in network science and learning how graph algorithms are used to solve science and engineering problems. R programmers may also find this book helpful as many algorithms also have R implementations.
https://rapidgator.net/file/bf53d0cd1cd751b10d0761abf2c944bf/
https://filestore.me/njtcm3wbm7ej
[/center]
- Distributed Graph Algorithms for Computer NetWorks
- Modern Graph Theory Béla Bollobás 1998
- C# Data Structures and Algorithms: Harness the Power of C# to build a diverse rang...
- Algorithms Python - Explains Algorithms With Beautiful Pictures Learn It Easy Bett...
- Algorithms Python Explains Algorithms With Beautiful Pictures Learn It Easy Better...
Permisos de este foro:
No puedes responder a temas en este foro.